037159u43
Copyright 2004 by the Genetics Society of America
Combining Mathematical Models and Statistical Methods to Understand and
Predict the Dynamics of Antibiotic-Sensitive Mutants in a Population of
Resistant Bacteria During Experimental Evolution
Leen De Gelder,* Jose´ M. Ponciano,† Zaid Abdo,† Paul Joyce,†
Larry J. Forney* and Eva M. Top*,1
*
Department of Biological Sciences, Initiative in Bioinformatics and Evolutionary Studies (IBEST), University of Idaho, Moscow,
Idaho 83844-3051 and †
Department of Mathematics, Division of Statistics, Initiative in Bioinformatics and Evolutionary
Studies (IBEST), University of Idaho, Moscow, Idaho 83844-1103
Manuscript received November 5, 2003
Accepted for publication July 28, 2004
Temporarily discontinuing the use of antibiotics has been proposed as a means to eliminate resistant
bacteria by allowing sensitive clones to sweep through the population. In this study, we monitored atetracycline-sensitive subpopulation that emerged during experimental evolution of
E. coli K12 MG1655carrying the multiresistance plasmid pB10 in the absence of antibiotics. The fraction of tetracycline-sensitive mutants increased slowly over 500 generations from 0.1 to 7%, and loss of resistance could beattributed to a recombination event that caused deletion of the
tet operon. To help understand thepopulation dynamics of these mutants, three mathematical models were developed that took into consider-ation recurrent mutations, increased host fitness (selection), or a combination of both mechanisms (fullmodel). The data were best explained by the full model, which estimated a high mutation frequency (! "3.11 # 10$5) and a significant but small selection coefficient (% " 0.007). This study emphasized thecombined use of experimental data, mathematical models, and statistical methods to better understandand predict the dynamics of evolving bacterial populations, more specifically the possible consequencesof discontinuing the use of antibiotics.
TODAY,numerouspathogenshavedevelopedresis- the absence of antibiotics (Godwin and Slater 1979;
tance to one or multiple antibiotics (Cohen 1992),
Helling
et al. 1981; Bouma and Lenski 1988; Modi
to such an extent it is called "an epidemic of microbial
and Adams 1991; Turner
et al. 1998; Dahlberg and
resistance" (Gibbons 1992). Aside from the constant
Chao 2003). Therefore, when plasmid-free segregants
search for new antibiotics that can be used against multi-
emerge, they might be expected to replace the resistant
drug-resistant pathogens, another strategy is to lower or
plasmid-containing population in the absence of selec-
discontinue the use of a specific antibiotic against which
tion for the plasmid. It has also been shown that the
resistance is widespread (Levy 1994; OTA 1995; WHO
elimination of resistance genes from a plasmid can lower
1995, 2003; Lenski 1997). This approach assumes that
the burden of that plasmid. During an evolution experi-
antibiotic resistance imposes a fitness cost to the host
ment under glucose-limited conditions, Godwin and
(Spratt 1996; Andersson and Levin 1999; Bjorkman
Slater (1979) observed that an initial population of
and Anderson 2000; Normark and Normark 2002)
Escherichia coli K12 that carried a multiple antibiotic
high enough for sensitive strains to have a selective
resistance plasmid was replaced by a tetracycline-sensi-
advantage relative to resistant ones in the absence of the
tive population that still carried a plasmid encoding
drug. Therefore, ending the use of a specific antibiotic
the other resistance determinants. Also Dahlberg and
would allow these sensitive strains to sweep through and
Chao (2003) and Turner
et al. (1998) detected differ-
replace the resistant population (Levin
et al. 1997). An
ent types of sensitive subpopulations in a multiresistant
important mechanism by which bacteria acquire antibi-
parent population. In a similar experiment with
E. coli
otic resistance genes is through conjugative transfer of
JA104 (pBR322&5), a plasmid variant that possessed a
plasmids on which they are encoded. Many authors have
2.25-kb deletion encompassing the tetracycline resis-
observed that the carriage of an antibiotic resistance
tance operon arose, which resulted in a 10–20% fitnessincrease for the host compared to carriage of the wild-
plasmid does indeed reduce the fitness of the host in
type plasmid (Modi
et al. 1991). The population car-rying this mutated plasmid increased steadily from 5 to99% between the 380th and 560th generation, repre-
1
Corresponding author: Department of Biological Sciences, 252 Life
senting a clear selective sweep. These studies suggest
Sciences S., University of Idaho, Moscow, ID 83844-3051.
that drug-sensitive mutants could replace the resistant
Genetics
168: 1131–1144 (November 2004)
L. De Gelder
et al.
population after ending antibiotic treatment. In con-
et al. 1966) in 50-ml flasks, which were shaken at 225 rpm at
30'. This medium supported a stationary-phase density of
trast, other reports have clearly shown that coevolution
!4 # 109/ml. Five lineages were founded from
E. coli
between plasmid-encoded genes and the host (Lenski
K12(pB10) and five from
E. coli K12::Km(pB10). Every 24 hr,
et al. 1994) or compensatory mutations (Schrag
et al.
39 (l of each population was transferred to fresh media. The
1997; Andersson and Levin 1999) may result in resis-
regime permitted 8 generations of binary fission every day.
tant strains being equally as fit as or even fitter than
At 100-generation intervals a 1-ml sample of each population
was mixed with 0.3 ml glycerol and archived at $80'. The
their sensitive counterparts.
founding populations were archived in the same way.
Mutations in bacteria that result in loss of resistance
Screening for tetracycline-sensitive clones: At weekly inter-
to a particular antibiotic are typically infrequent. The
vals from day 14 on, a sample from three lineages of each
frequency of spontaneous mutations has been estimated
K12(pB10) and K12::Km(pB10) was plated on LB and colonies
to be in the range of 10$9–10$10 per base pair and per
were picked onto LBTc, LBAmx, and LB. Colonies that scored
Tc$Amx)Sm) were archived at $80'. From both archived
generation (Drake 1991; Andersson and Hughes 1996;
start cultures,
E. coli K12(pB10) and
E. coli K12::Km(pB10), a
Drake
et al. 1998) and only a fraction of these mutations
sample was plated on LB, and 1000 colonies were picked onto
will convert a resistant phenotype into a sensitive one.
LBTc, LBAmx, and LB. Samples from the frozen cultures that
Due to these low frequencies and the lack of direct detec-
were archived every 100 generations were plated onto LB and
tion of antibiotic-sensitive clones, such clones that arise
colonies (see Table 1 for sample size) were picked onto LBTc,
LBAmx, and LB.
within a resistant population are usually observed only
Plasmid extraction and restriction enzyme analysis: Plasmids
once they sweep through the population due to a fitness
were extracted from cells grown in LBTc or LBAmx using the
advantage in the absence of the antibiotic.
QIAprep spin miniprep kit according to the manufacturer's
In this study, we monitored the loss of antibiotic resis-
instructions (QIAGEN, Valencia, CA) and digested with
Hin-
tance in
E. coli K12 populations that carried the multire-
dIII or
NotI (Invitrogen, Carlsbad, CA). Samples were loaded
on a 0.8% agarose gel and run for !16.5 hr at 30 V. The
sistance IncP1-* plasmid pB10 during a long-term evolu-
gels were stained with ethidium bromide and the bands were
tion experiment in a medium without antibiotics. Our
visualized by UV light.
goal was to identify the molecular basis of the tetracy-
PCR and sequencing: PCR was used to assess the occurrence
cline sensitivity observed in the mutant population and
of recombination between the direct repeats that flank the tetra-
to develop a mathematical model based on the data
cycline-resistance operon. These primers (forward, 5+-GACGG
CGGCCTGGAGACAAGTC; reverse, 5+-TTTGCTCGGTGCCCT
that helped explain the observed population dynamics,
TTCGGGTAA) targeted the regions on the plasmid (Schlu¨ter
taking into account the associated mutation frequency
et al. 2003) just outside the direct repeat sequences as de-
and selection coefficient. This model was further used
scribed previously (Flores
et al. 2000). If no recombination
to generate predictions concerning the effects of cycling
has taken place and the tetracycline-resistance operon is still
antibiotics on the decline of resistance levels.
present in the plasmid, the annealed primers would be sepa-
rated by an !6.4-kb stretch and the PCR reaction would not
continue. If recombination has occurred and the tetracycline-
resistance genes have been lost, the primers would be sepa-
MATERIALS AND METHODS
rated by !900 bp and a PCR product of that size would be
formed. PCR products were cleaned with the MiniElute PCR
purification kit according to the manufacturer's instructions
Media: Luria-Bertani broth (LB) medium and M9 minimal
medium were prepared according to Sambrook and Russel
To sequence the 900-bp fragment, 2 (l of PCR product was
(2001). Unless otherwise stated, antibiotics were used in the
mixed with 1 (l of either the forward or the reverse primer
following concentrations: 10 mg/liter tetracycline (Tc), 50
and 3 (l ddH2O and put at 95' for 5 min, after which it was
mg/liter kanamycin (Km), 75 mg/liter amoxicillin (Amx),
cooled down to 50'. Then, 4 (l of BigDye Terminator cycle
and 40 mg/liter streptomycin (Sm).
sequencing ready reaction mix (Version 3.0; Perkin-Elmer,
Bacterial strains and plasmid: The bacterial strains used
Foster City, CA) was added. Cycle sequencing conditions (Pel-
were
E. coli K12 MG1655 (ATCC 47076) and a Km-resistant
tier Thermal Cycler-200; MJ Research, Reno, NV) were as
variant (K12::Km) obtained by conjugation of
E. coli K12 with
follows: 95' for 30 sec, 50' for 20 sec, 60' for 4 min, with a
E. coli S17/1 !pir (pUTminiTn5Km) and selection on M9 )
total of 44 cycles performed. The sequencing products were
0.4% lactose ) Km. The 64.5-kb plasmid pB10 was isolated
cleaned with the DyeEx 2.0 spin kit (QIAGEN) for dye-termi-
from the bacterial community of a wastewater treatment plant
nator removal, followed by vacuum centrifuging for 10 min,
in Germany (Dro¨ge
et al. 2000) and has been sequenced
after which 10 (l formamide was added and the samples were
(Schlu¨ter
et al. 2003). It has been identified as a self-transmis-
put at 96' for 3 min. They were kept on ice before running
sible broad-host-range IncP-1* plasmid and it mediates resis-
on a POP6 polymer on a 3100 DNA automated capillary se-
tance against the antibiotics tetracycline, amoxicillin, sulfon-
quencer (Applied Biosystems, Foster City, CA).
amide, and streptomycin and against mercury ions. The
Electroporation: To obtain ancestral strains carrying
plasmid was transferred from its original host
E. coli DH5, to
evolved, mutated pB10 plasmids,
E. coli K12 cells were pre-
K12 and K12::Km through conjugation and selection on BBL
pared for electroporation according to Enderle and Farwell
MacConkey Agar supplemented with 15 mg/liter Sm and
(1998). Approximately 200 ng of plasmid DNA was added to
each of the cell suspensions and the mixtures were brought
Evolution experiment: Experimental evolution of 10 inde-
into electroporation cuvettes (1 mm gap, 100 (l volume; Ep-
pendent lineages was accomplished by serial batch culture of
pendorf, Hamburg, Germany) and electroporated at 2.5 kV
each lineage for 500 generations in 10 ml M9 with 2 g/liter
(25 (F, 200 -). The suspensions were then immediately trans-
glucose as sole carbon source and 5 ml/liter stock E (Stanier
ferred to SOC medium (Sambrook and Russel 2001), incu-
Modeling Antibiotic Resistance Loss
bated for 1 hr at 37', and plated on selective media after which
lk (*0 , %, !) "
the presence of the appropriate plasmid was confirmed.
mlk )
nlk
Competition experiments: Competition experiments were
performed by mixing overnight cultures of the two competi-
Solving this recursion (appendix b) results in the following
tors grown in M9 ) 2 g/liter glucose ) 5 ml/liter stock E in
general equation:
a 1/100 ratio. For each experiment, six to eight replicate cultures
*
lk (*0 , %, !)
were tested. The cultures were transferred every 24 hr into
fresh media (39 (l inocula into 10 ml of fresh media). After
0(2% $ (1 $ !)) ) !(1 $ *0)(2
lk% $ (1 $ !)
lk )
two transfers, the ratio of the two competitors was determined
0(2% $ (1 $ !)) ) !(1 $ *0 )(2
lk% $ (1 $ !)
lk) ) (1 $ !)
lk(1 $ *0 )(2% $ (1 $ !))
by selective plating. Using these data, the relative fitness (
W)
was calculated as described previously (Lenski
et al. 1991).
Equation 5 assumes that both mutation (at rate !) and selec-
Subsequently, the selection coefficient,
s "
W $ 1 (Lenski
et
tion of mutants (represented by the selection coefficient %)
al. 1991), was calculated and a
t-test was performed to deter-
affect the population dynamics. This equation is readily inter-
mine if the
s-value was significantly different from zero. A
t-test
pretable. The denominator is composed of three parts:
was also performed to detect significant differences between
2
lk%*0(2% $ (1 $ !)) represents the growth of initial mutants,
!(1 $ *0 )(2
lk% $ (1 $ !)
lk ) represents the formation and
growth of new mutants during evolution, and (1 $ !)
lk(1 $*0 )(2% $ (1 $ !)) represents the growth of TcR cells. The
Mathematical models and statistical methodology
numerator involves the first two terms and describes the forma-
tion and growth of mutants. When there is no fitness differ-
Modeling the experimental evolutionary process: The ex-
ence between the mutants and the wild type we can set the
perimental evolutionary process described above consisted of
selection coefficient % to zero. Equation 5 becomes
daily growth periods, wherein the populations multiplied in
a neutral environment for
l generations (
l " 8), interspersed
*
lk (*0 , 0, !) " 1 $ (1 $ *0 )(1 $ !)
lk.
by daily bottlenecks of 39 (l/10,000 (l. We define a cycle as
Then the increase in fraction of mutants in the population is
the combination of a growth period and a bottleneck and a
due solely to mutation. When there is no mutation effect, !
neutral environment as one that does not contain any antibiot-
can be set equal to zero, and the relative increase in mutants
ics. The growth period of any cycle might encompass the event
is modeled only by the selective growth advantage:
of a deletion mutation occurring at random and resulting in
a plasmid without
tet operon. We refer to cells with a mutated
plasmid as mutants.
lk (*0 , %, 0) "
*02
lk(1)%) ) 2
lk(1 $ *0 )
We assumed the mutation process to be unidirectional;
i.e.,
the cells can lose the Tc resistance but cannot gain it. We
Modeling the sampling process: Samples were taken every
denote the average number of mutants at a certain time
t by
100 generations and diluted and plated to screen for tetracy-
mt and the average of the remaining wild-type cells in period
cline-sensitive clones. The used sample sizes, from 42 to 1000
t by
nt. Therefore, the total population at time
t is the sum
colonies, were very small compared to the bottleneck imposed
of
mt and
nt. At any generation, mutants increase due to (i)
(!107 transferred cells) and can result in a magnification of
growth of the mutants from the previous generation, which
stochastic effects (Joyce
et al. 2005);
i.e., the numbers of mu-
occurs at a rate 21)% with % being the selection coefficient
tants observed on the basis of such a sampling method might
and (ii) mutation of wild-type cells at rate !. The following
fluctuate merely due to the randomness of this sampling pro-
recursive equation captures the average number of mutants
cess. We call such an effect "observational error" and use a
at time
t:
stochastic model in the analysis of this process. A stochastic
model that fits such a process is a binomial sampling process.
mt " 21)%
mt$1 ) 2!
nt$1.
In a sample of size
Dk, taken at the
kth day, each individual
has a probability *
lk of being a mutant and 1 $ *
lk of being
Using the no-back-mutation assumption, the average number
a wild type. If *
lk is small we can approximate this model
of nonmutants at any time
t is given by
to a Poisson model with parameter
Dk*
lk, representing the
expected number of mutants in a sample. Letting this number
nt " 2(1 $ !)
nt$1.
of mutants from culture
i at the end of day
k,
Sik, be a random
variable, then
Sik is modeled by
The average fraction of mutants available at any time
t is
Sik ! BIN(
Dk, *
lk) ! POI(
Dk*
lk)
t (*0 , %, !) "
t )
nt
ik "
sik ) "
*0 represents the fraction of mutants that might already be
available at the start of the experiment.
Statistical analysis: Equation 8 presents the main model
Every cycle
k encompasses
l generations. Joyce
et al. (2005)
used in our data analysis and provides the link between the
showed that for the purposes of statistical analysis one can
mathematical modeling and the statistical analysis, which pro-
assume that the fraction of mutants at the end of each cycle,
ceeded in three stages. First, the model was fitted to the data
*
lk , is deterministic and unaffected by the bottleneck. [While
using a maximum-likelihood approach. We estimated the mu-
the results of Joyce
et al. (2005) were derived for the special
tation rate ! under the mutation-alone model and estimated
case where % " 0, it can be shown both analytically and by
the selection coefficient % under the selection-alone model.
simulation that the effect of the bottleneck on the variation
Both parameters (!, %) were jointly estimated under the gen-
of the process is strongest when % " 0. So even in the case
eral (mutation and selection) model. Next, we determined
where the highest amount of variation due to the bottleneck is
whether any of the models adequately described the data using
expected, the effect is still negligible.] Time can be measured
an absolute goodness-of-fit test and a parametric bootstrap
using
lk to reflect the cyclic behavior of the system. Equation
approach. Finally, we assessed the relative fit of the model by
comparing the simpler cases (mutation alone, selection alone)
L. De Gelder
et al.
to the general model by using a likelihood-ratio test and a
various time points as by taking samples of size
n for each of
parametric bootstrap approach.
r replicated experiments. Since replicating the experiment is
Parameter estimation: To estimate the parameters !, %, and
more time consuming and expensive than simply taking larger
*0, we used the method of maximum likelihood (Bain and
samples, one might think that our article argues against repli-
Engelhardt 1991; Rice 1995). The probability of a data set
cation. However, one has a much better chance of detecting
S " {
sik} given the model
M is referred to as the likelihood
violations of model assumptions if one replicates the experi-
of that data set under the model. Using the Poisson model
ment. Our program can be downloaded at the website http://
approximation (Equation 8) and assuming that we have
rk
independent replicates per cycle
k and that these replicates
user's manual that can be downloaded along with the program
are independent across cycles, the likelihood of the data set
provides detailed information on the effect of departures from
S given the model is
model assumptions and how to test for these departures.
Comparing the different models: We compared the mutation-
alone and the selection-alone models to the full model. The
Dk, !, %, *0) " " "
ik "
sik Dk , !, %, *0 )
likelihood-ratio test statistic needed to compare the mutation-
alone model to the full model is given by
" " "
M$
F "
L(*ˆ0, %ˆ, !ˆ)
.(*ˆ0, %ˆ, !ˆ)
Multiple methods are available for maximizing the likelihood
of the data. We used the Nelder-Mead algorithm (Nelder
where
L is the likelihood function as defined in Equation 9,
and Mead 1965) implemented in both MatLab6.5 and R1.6.2
(*ˆ0, %ˆ, !ˆ) are the MLEs, and .
M(*ˆ0, !ˆ) and .(*ˆ0, %ˆ, !ˆ) are
(GNU license). The resulting estimated parameters are re-
the absolute goodness-of-fit likelihood test statistics (Equation
ferred to as the maximum-likelihood estimates (MLEs). Pro-
10) for the mutation-alone and the full models, respectively.
grams, using MatLab6.5 and R1.6.2 (http://www.r-project.
Taking the natural log the following equation was obtained:
org), were developed for estimation. These programs are avail-
able through the web under http:/ www.webpages.uidaho.edu/
$2 ln(.
M$
F ) " $2 ln(.
M (*ˆ0 , !ˆ)) ) 2 ln(.(*ˆ0 , %ˆ, !ˆ)).
Absolute goodness of fit: The absolute goodness-of-fit test for
each of the three models considered is based on the null
A similar setup for the comparison of the selection-alone
hypothesis that the data are Poisson distributed with
E(
Sik) "
model to the full model results in
Dk*
lk against the alternative that each
Sik is Poisson distributed
with a different mean (
ik (Rice 1995). The associated likeli-
2 ln(.
S$
F) " $2 ln(.
S(*ˆ0, %ˆ)) ) 2 ln(.(*ˆ0, %ˆ, !ˆ)).
hood-ratio test (LRT) statistic . is derived in appendix c and
A 2000-replicates parametric bootstrap was also used to gener-
ate the distribution of the LRT. MatLab6.5 and R1.6.2 (http://
. " " " #
Dk*ˆ
lk$
sike$
Dk*ˆ
lk)
sik,
www.r-project.org) programs in the S language were also devel-
oped for this analysis and are also available at http://www.web
where *ˆ
lk is the estimated proportion of mutants at the end
of the
kth cycle, computed by replacing the null-model param-
eters by the MLEs in the solution of Equation 4. The solution
equation needed changes depending on which model is tested
(see Equations 5–7). By the invariance principle of the MLEs
(Rice 1995), *ˆ
lk is also a MLE. Therefore, this estimate is
Experimental results: Detection of tetracycline-sensitive
different for each of the three absolute goodness-of-fit tests.
clones: We sought to monitor the loss of antibiotic resis-
Taking the natural logarithm of the likelihood-ratio statistic
tance in an
E. coli K12 population carrying the multire-
. results in the following familiar format:
sistance plasmid pB10 during a long-term evolution ex-
ˆ
lk $
sik) .
periment in a medium without antibiotics. To do this,
ik ln#
sik
10 independent lineages were evolved for !500 genera-
We used a parametric bootstrap (Efron and Tibshirani 1993)
tions in serial batch cultures. Loss of resistance to tetra-
to estimate the distribution of this $2 ln . test statistic. For
cycline or amoxicillin was monitored weekly in 6 of the
each case, 2000 data sets were simulated under the Poisson
10 lines starting from day 14, as well as in the 2 founding
distribution of the null model, taking its MLEs to be the true
cultures. Tetracycline sensitive (TcS) clones were de-
parameter values. Then we estimated the MLEs for each of the
simulated data sets, thus generating a sampling distribution for
tected in the founding cultures (0.15%) and their frac-
these MLEs. This sampling distribution was used to construct
tion gradually increased on average over 500 genera-
confidence intervals and confidence regions for the MLEs
tions to !6% (Joyce
et al. 2005). All but one of the TcS
associated with the actual data. Applying Equation 11 to each
clones were still resistant to amoxicillin and streptomy-
of the simulated data sets and their associated MLEs we gener-
cin, indicating a very high plasmid maintenance. Since
ated a distribution of the $2 ln . test statistic. The proportion
of times that the simulated $2 ln . values were greater than
the frequency of the TcS clones was rather variable,
the $2 ln . calculated using the actual data set resulted in a
probably due to the small sample size (maximum of 52
P-value. This proportion is an estimate of the probability that
clones per culture per time point), a new sampling
a LRT value greater than or equal to the one observed would
scheme that fixed the relative error rate at 0.21 was
actually occur given that the hypothesized model is true.
derived (Joyce
et al. 2005). This allowed us to more
It is interesting to note that if the above model assumptions
are accurate, then one can gain the same amount of informa-
precisely determine the fraction of the TcS mutants in
tion from a single replicate with samples of size
r #
n at
all 10 available cultures that were archived every 100th
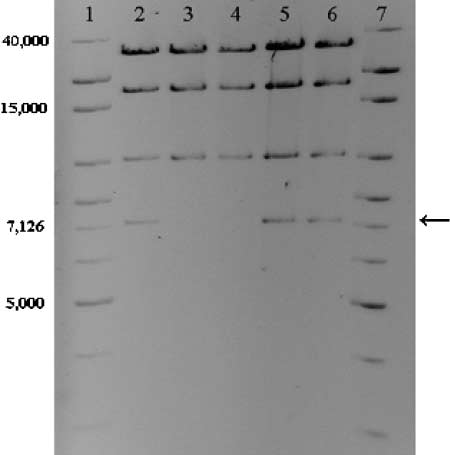
Modeling Antibiotic Resistance Loss
Fraction of tetracycline-sensitive mutants found in 10 parallel and independent lineages over 504 generations,
as well as the predicted fractions under the three models
Observed fractions
Predicted fractions
WT1: WT2: WT3: WT4: WT5: Km1: Km2: Km3: Km4: Km5:
0.0015 0.0013 0.0029 0.0010
0.010 0.000 0.000 0.010 0.005 0.005 0.010 0.000 0.010 0.005 0.0053 0.0063 0.0059 0.0107
0.010 0.019 0.019 0.000 0.010 0.010 0.010 0.019 0.010 0.000 0.0106 0.0137 0.0113 0.0196
0.015 0.060 0.075 0.015 0.030 0.015 0.030 0.015 0.090 0.030 0.0373 0.0266 0.0229 0.0292
0.058 0.058 0.000 0.000 0.058 0.096 0.077 0.038 0.058 0.038 0.0481 0.0453 0.0436 0.0379
0.045 0.136 0.091 0.023 0.068 0.000 0.023 0.114 0.114 0.068 0.0682 0.0766 0.0853 0.0473
Each independent lineage is labeled as either WT1–5 [E. coli K12(pB10) lineages] or Km1–5 [E. coli K12::Km(pB10) lineages].
k denotes the cycle and l the number of generations per cycle (l " 8). Dk is the number of clones screened and sik (i " 1, 2, 3,
. . 10) is the observed fraction of mutants for each replicate i at cycle k. M & S, mutation and selection model; S, selection-
alone model; M, mutation-alone model.
generation. As shown in Table 1, the fractions of mu-
lated from a clone obtained at generation 500 [yielding
tants observed in these new samples showed a clear
K12::Km(pM57)], and this strain was competed against
increasing trend over time. All TcS clones obtained were
the ancestral host with ancestral plasmid K12(pB10).
still resistant to amoxicillin, indicating that they had not
The selection coefficient (s-value) was 0.0273, which was
lost the entire plasmid.
not significantly different (P " 0.974) from the s-value
Characterization of the plasmids of tetracycline-sensitive mu-
(0.0271) of the control experiment that detected fitness
tants: To examine whether the loss of tetracycline resis-
differences due to the chromosomal marker [K12::Km
tance was due to deletions or rearrangements in the
(pB10) vs. K12(pB10)]. The results of these pairwise
plasmid, restriction fragment length polymorphisms
competition experiments suggest that deletion of the
(RFLPs) of the plasmids were determined. Comparison
plasmid fragment containing the tet operon did not
of these RFLPs with the theoretical restriction map of
measurably increase the host fitness. Therefore it ap-
plasmid pB10 (Schlu¨ter et al. 2003) showed that the
plasmids of all 73 sensitive clones examined lacked a7057-bp HindIII fragment, whereas the patterns of ran-domly picked TcR clones were not different from thatof the ancestral plasmid (Figure 1). A NotI restrictionanalysis performed on a few TcS clones showed thatthe 13,920-bp NotI fragment was not present in theirplasmids. Analysis of the plasmid sequence revealed thatthe differences in RFLPs arose as the result of deletion ofa 5.5-kb region that included the tetracycline-resistanceoperon (tetA, tetR) and was flanked by two direct repeatsof 863 bp. This suggests that the sensitivity to tetracyclinein the mutants was due to deletion of the tet operon byrecombination between the direct repeats. To furtheranalyze this deletion event, this region of plasmids from18 mutants from six different lineages was amplifiedusing primers described above and sequenced. All PCRproducts obtained from the sensitive clones showedidentical DNA sequences, in which only one of the directrepeats was still present and the sequence in betweenthe repeats was absent. This indicated that the deletionof the tetracycline-resistance operon was caused by a
Figure 1.—HindIII RFLP patterns of ancestral and evolved
recombination event between the two flanking direct
plasmids. Lanes 1 and 7, 1-kb extended ladder, 5 (g and 10(g, respectively; lane 2, ancestral pB10; lanes 3 and 4, plasmid
DNA from TcS mutants; lanes 5 and 6, plasmid DNA from
Competition experiments: To investigate the effects of
evolved TcR clones. Arrow indicates missing band in TcS mu-
the mutated plasmid on host fitness, the ancestral host
tants. Colors were inverted and brightness and contrast were
K12::Km was transformed with a mutated plasmid iso-
adjusted to enhance the quality of the image.
L. De Gelder et al.
peared that the increase in frequency of the TcS mutants
could not be attributed to a selective advantage.
Modeling and statistical analysis: Parameter estimates
and confidence intervals: A mathematical model was devel-
oped to capture the observed dynamics of the TcS mu-
tants in the population. Overall, the data analysis strongly
indicated that both selection and frequency of muta-
tion, as described by the full model, seemed to affect the
population dynamics of the mutants. Neither selection
alone nor mutation alone could explain the observed
patterns of population increase as well as the full modeldid. Using the full model, the selection coefficient %
was estimated to be 0.00699 and the rate of mutation
! was estimated to be 3.11 # 10$5/generation (Table
2). The MLEs and their confidence intervals were sig-
nificantly distinct from zero in each of the three cases.
Further corroboration of this conclusion is indicated in
Figure 2, which presents the bootstrapped joint 94.8%
confidence region for the mutation rate ! and the selec-
tion coefficient % of the full model. This region excludes
zero, indicating that the parameter estimates are sig-
nificant positive quantities. The joint confidence regioncan be thought of as an inverted likelihood-ratio test:
it is the set of all the parameter values consistent with
the hypothesized model. The MLE of the selection coef-
ficient increased by 142% in the selection-alone model
compared to its estimated value using the full model.
This increase compensates for the absence of mutation
in this model. When considering mutation alone in the
percentiles, cient;
model, the MLE of the mutation rate increased by 303%
from its value calculated using the full model. The third
parameter, the initial fraction of mutants *0, was esti-
mated under each model and also found to be signifi-
cantly different from zero (Table 2).
Testing the models: Utilizing the MLEs and each of the
three models, we calculated the average proportion of
the mutants at the end of each of the sampled genera-
tions (Table 1). These predictions were used to con-
struct Figure 3. The closer the dispersion of the circles
around the curves in Figure 3A or the diagonal solidline in Figure 3B, the better the fit. On the basis of this,
both the mutation and the selection and the selection-
alone models seem to be fitting the data better than
the mutation-alone model, which drastically underesti-
mated the mean mutant proportions toward the end of
the experiment. Table 3 introduces the bootstrapped
likelihood-ratio results for both the absolute goodness-
of-fit tests and the model-comparison tests. The P-value
of the absolute goodness-of-fit tests confirmed that the
proposed full and selection-alone models fit the data
quite well, while the mutation-alone model (P-value "
0.0065) does not. The P-values associated with testing
the selection-alone and the mutation-alone models againstthe full model were 0.0025 and 0, respectively. This
provides clear statistical evidence that the full model is
significantly better than the other two simple models.
This important result suggests that both selection and
Modeling Antibiotic Resistance Loss
Figure 2.—Plot of the joint 94.8% bootstrapped confidence region for both the mutation rate ! and the selection coefficient
%. The solid diamonds represent the joint parameter estimate of the mutation rate ! and the selection coefficient %.
mutation, together, played a significant role in the ob-
totic theory and provides the correct sampling distribu-
served evolutionary process. Figure 4 provides a visual
tions under the model assumptions.
examination of the absolute goodness-of-fit results.
The bootstrap vs. the asymptotic theory: Under certain
regularity conditions that guarantee that the MLEs are
asymptotically normally distributed, the $2 ln(.) test
We observed a gradual increase in the frequency of
statistic (plotted in Figure 4 for each model) is /2 distrib-
a tetracycline-sensitive subpopulation during experi-
uted in the limit as the sample size increases (Bickel
mental evolution of E. coli K12(pB10) in the absence
and Doksum 1977; Self and Liang 1987; Bain and
of antibiotics and showed that this phenotypic change
Engelhardt 1991). Accordingly, it is tempting to use
was caused by deletion of the plasmid-encoded tet op-
the asymptotic theory rather than the bootstrap in hy-
eron. The extent to which these tetracycline-sensitive
pothesis testing; the /2 distribution is readily tabulated
mutants sweep through a population and replace the
and only degrees of freedom have to be known to find
residing resistant clones was determined by the muta-
the P-values. However, our simulations definitely showed
tion rate and the selection coefficient associated with
that the asymptotic /2 distribution was not valid (results
this phenotypic and genotypic change. Therefore we
not shown). The use of the /2 distribution resulted in
estimated the values of these two parameters by mathe-
overly conservative incorrect P-values and hence a
matically modeling the dynamics of the tetracycline-
higher tendency to reject the null when true (higher
sensitive subpopulation. Such a modeling approach has
type I error). Moreover, the selection-alone model lends
not been used previously in similar studies (Godwin
itself to a special boundary problem when the mutation
and Slater 1979; Turner et al. 1998; Dahlberg and
rate is set to zero as the mutation rate cannot be nega-
Chao 2003), where sweeps of antibiotic-sensitive mu-
tive. The asymptotic distribution in this case is not the
tants were observed during experimental evolution. We
regular /2 distribution under the general theory but a
report here a very high mutation frequency in agree-
tighter mixed distribution (Self and Liang 1987). The
ment with the nature of the mutation and a low selection
parametric bootstrap approach does not rely on asymp-
coefficient that could not be detected by means of com-
L. De Gelder et al.
dynamics of the tetracy-
cline-sensitive mutants. (A)
Time course of the observed
(!) and predicted (—) av-
erage fractions of mutants
under each of the three
mathematical models. (B)
Ratios of observed vs. pre-
dicted fractions of mutants
under each model. The
solid lines indicate identical
observed and predicted val-
ues. All replicate and aver-
age values are reported in
petition experiments. Rejecting both the mutation-alone
10$5/generation. This value corresponds fairly well with
and selection-alone models with high statistical signifi-
the estimate we obtained experimentally by determining
cance in favor of the full model (mutation and selec-
the fraction of TcS mutants in a colony grown overnight
tion) highlighted the strong impact of both mutation
on LB agar, founded by a cell from an overnight culture
and selection together on the dynamics of the sensitive
of K12(pB10) in LBTc. Out of 941 clones screened within
the colony, 3 had lost the tet operon after !26 genera-
As confirmed by sequencing, the loss of tetracycline
tions [" log2(108)], yielding a deletion frequency of
resistance in all sensitive clones examined can be attrib-
!1.2 # 10$4/generation. This high mutation frequency
uted to a recombination event between two direct re-
is due to the specific nature of the mutation, namely a
peats present on the plasmid that are flanking the tet
recombination event. In general, recombination fre-
operon. According to the parameter estimates of the
quencies have been reported from as high as 10$3 to as
"mutation and selection" model, the frequency of the
low as 10$11 (Dianov et al. 1991; Mazin et al. 1991;
deletion of the tet operon was determined to be 3.11 #
Lovett et al. 1994; Bi and Liu 1996) depending on thelength of the direct repeats and the distance betweenthem. Considering the long direct repeats (863 bp)
flanking the tet operon and the large fragment being
Parametric bootstrap maximum-likelihood-ratio tests
deleted (5.5 kb), the estimated high mutation frequencyof 3.11 # 10$5/generation is in agreement with the
range of values previously described. Thus, in the ab-sence of antibiotics, this fragment containing the tet
Mutation and selection
operon was systematically lost at a fairly high rate, caus-
ing a tetracycline-sensitive subpopulation to emerge and
Selection vs. mutation and selection
to expand initially according to a linear pattern. In the
Mutation vs. mutation and selection
absence of selection, continuous deletion events wouldresult in a steady increase of the TcS population as de-
In the first three rows, the P-value is an estimate of the
probability that a $2 ln . value greater than or equal to the
scribed by the "mutation-alone" model (Figure 3A).
one observed would actually occur given that the hypothesized
In addition to deletions of resistance genes from a
model is true. For the last two rows, it gives an estimate of
plasmid, the loss of the entire plasmid itself could also
the probability that a difference in model scores would be as
cause resistance to decline in a bacterial population.
large as or larger than the one actually seen if the data came
from the selection-alone and mutation-alone models, respec-
Although the fitness cost of carrying the plasmid pB10
was estimated to be 0.02 (our unpublished data), only
Modeling Antibiotic Resistance Loss
Figure 4.—Pictorial repre-
sentation of the bootstrap like-
lihood-ratio test (LRT) out-
comes for each mathematical
model. The plotted density is
the bootstrap sampling distri-
bution of the LRT, $2 ln .
The P-value (pBoot) is the area
under the curve to the right of
the dashed vertical lines (corre-
sponding to the $2 ln . ob-
served value). It represents the
probability that a LRT value
greater than or equal to the
one observed would actually
occur given that the hypothe-
sized model is true.
one plasmid-free segregant out of 7086 screened clones
introduces considerable variation between replicate
was detected during the 500 generations of evolution.
competition experiments, lowering the statistical power
This indicates that the plasmid was very stably main-
of the test. Ideally, competition experiments are con-
tained, which is a known characteristic for IncP-1* plas-
ducted only for one growth cycle (Lenski et al. 1991;
mids (Thomas 2004), or that plasmid-free segregants were
Dahlberg and Chao 2003), although an increased sen-
rapidly reinfected by plasmid-carrying cells (Thomas
sitivity can be obtained by measuring fitness over several
2004). A similar observation has recently been made
cycles (Dahlberg and Chao 2003). However, in the
by Dahlberg and Chao (2003), where during 1100
latter case, mutations, not just those at the tet locus but
generations no plasmid-free cells were ever detected in
also in the chromosome, could be a confounding factor
R1- or RP4-bearing populations under antibiotic-free
that can influence the competition process in the long
conditions, although a large fitness cost for carrying the
run. Both the mutant and ancestral populations would
plasmid had been determined. These results suggest
continue to evolve during a long competition experi-
that even though resistance plasmids confer a cost to
ment and one would have a difficult time trying to
their host, plasmid-free segregants will not necessarily
demonstrate that the differences in fitness were due to
form and thus cannot sweep through the population,
the original mutation, i.e., the tet deletion. In our study
thereby lowering the level of resistance. It also follows
competition experiments were already run for three
that the loss of one resistance gene on a multiresistance
growth cycles, or 24 generations, and extending this pe-
plasmid such as pB10 may still leave the host with other
riod is thus not recommended. In our view the strength
plasmid-encoded resistance determinants.
of the modeling effort lies in its ability to produce a better
In competition experiments, we did not detect a sig-
way of detecting small fitness differences between evolved
nificant fitness advantage for the ancestral E. coli K12
and ancestral strains compared to a competition experi-
carrying a deleted evolved plasmid compared to car-
ment. This was achieved by reproducing the observed
rying the ancestral plasmid. On the other hand, the
patterns on the basis of hypothesized processes (mutation
parameter estimate of the selection coefficient under
and selection acting together) and provided appropriate
the mutation and selection model was determined to be
explanations and predictions. Furthermore, the proposed
0.007 and statistically significantly different from zero.
processes were adequately tested statistically.
Selection coefficients of 01% are considered to be quite
The small cost imposed by the tetracycline-resistance
low and difficult or even impossible to detect with statis-
determinant encoded on plasmid pB10 may be explained
tical significance in a competition experiment (Levin
by the presence of the repressor TetR, which represses
et al. 1997; Andersson and Levin 1999). This is proba-
expression of tetA in the absence of tetracycline. This
bly due to experimental error and stochasticity, which
is in agreement with previous studies, which concluded
L. De Gelder et al.
Long-term predictions for the decline of the tetracycline-resistant population
Mutation and selection
Time (yr) to replace
Time (yr) to replace
Predicted time required to displace the resistant population to 1/1000 (99.9% sensitive) and to 1/1,000,000
(99.9999% sensitive) under the mutation-alone model and the mutation and selection model is shown. 1st CL
and 2nd CL represent the limits of a 95% confidence interval. MLE is the maximum-likelihood estimator.
that deletion of a constitutively expressed plasmid-
This is quite different from our approach where we
encoded tet operon drastically increased host fitness in
formally fitted the model to the data.
the absence of tetracycline (Modi et al. 1991), but that
In response to the widespread emergence of multire-
carrying an inducible tetracycline-resistance operon im-
sistant pathogens, a number of measures have been
poses essentially no burden (Lee and Edlin 1985; Ngu-
proposed to reverse this trend, including cycling differ-
yen et al. 1989).
ent antibiotics and reductions of antibiotic use (Levy
The rigorous combination of mathematical model-
1994; OTA 1995; WHO 1995; Bonhoeffer et al. 1997;
ing, statistical methods, and biological experiments, ap-
Lenski 1997; WHO 2003). It is believed that this will
plied in this work, is rarely seen in our field. Lenski et
allow sensitive clones with a selective advantage to sweep
al. (1991) used a regression framework to fit an evolu-
through the population and thereby eliminate their re-
tionary model to their data, including only selection,
sistant counterpart (Levin et al. 1997) as seen by Modi
and compared the differences between the fitnesses of
et al. (1991) in evolution experiments. Our study shows
their bacterial lineages using ANOVA. They also intro-
that such elimination of drug resistance proceeds very
duced models to explain the dynamics of their experi-
slowly when the cost of resistance is very small. Yet even
ments that included the mutation rate although did not
such a small selective advantage is of great importance
estimate parameters for these models using the data.
in the long run, as shown by comparison of predictions
Instead, they used their fitness estimate and an estimate
made by the mutation-alone and the full model (Table
of the effective population size to infer the mutation
4). The mutation-alone model predicts that even with
rate associated with some of these models. Austin et
the high estimated mutation rate, replacing 99.9% of
al. (1999) have fit a model to data on occurrence of
the resistant population with TcS mutants would take
resistance levels in hospitals and estimated the corre-
between 21 and 32 years at eight generations per day.
sponding parameters, using weighted least squares, but
However, when including the small drug-resistance cost
they did not thoroughly evaluate its goodness of fit, nor
(% " 0.007) in the mutation and selection model, such
did they test the significance of their parameters. In
a replacement of 99.9% would take only between 0.55
contrast, we used the method of maximum likelihood
and 1.83 years. To reach the same replacement level in
(Fisher 1922) to fit our models and estimated the asso-
5 weeks on average, the resistance cost should be at
ciated parameters on the basis of the experimental data.
least 0.06, which is realistic for a constitutively expressed
Likelihood-ratio tests and the parametric bootstrap were
tetracycline operon on a high-copy-number plasmid
used to evaluate the goodness of fit and to quantify
(Modi et al. 1991), but not for an induced resistance
our confidence in these models. Many studies present
on a low-copy-number broad-host-range plasmid. Our
mathematical models that describe evolutionary pro-
findings thus indicate once again that the cost of resis-
cesses involving bacteria (Bonhoeffer et al. 1997; Levin
tance is the key factor to successfully displace antibiotic-
et al. 1997; Otto and Whitlock 1997; Bergstrom et
resistant populations with sensitive ones (Spratt 1996;
al. 2000; Wahl and Krakauer 2000; Wahl and Gerrish
Lenski 1997; Levin 2001), and that the time required
2001; Johnson and Barton 2002; Martiel 2002; Wahl
for this, after ending the drug treatment, may be very
et al. 2002). In general, such studies focus more on
long. Moreover, even with 0.0001% of the resistant pop-
presenting the model itself and often do not fit experi-
ulation still present in the environment, which would
mental data to the model. In the few cases where these
take !1.3 years (Table 4), the reintroduction of the
studies involve data (Levin et al. 1997; Wahl and Kra-
antibiotic will cause the resistant bacteria to ascend to
kauer 2000; Wahl et al. 2002), these are usually used
high frequencies again, at a much higher pace than the
to compare against the results of the suggested model.
original decline (Levin et al. 1997; Austin et al. 1999;
Modeling Antibiotic Resistance Loss
of Health (NIH) grant P20 RR 16448 from the Centers of Biomedical
Heinemann et al. 2000). Thus, as a guideline for design-
Research Excellence Program of the National Center for Research
ing new antibiotics, our results support the statement
Resources. Its contents are solely the responsibility of the authors and
made earlier (Bjorkman and Anderson 2000) that the
do not necessarily represent the official views of NIH. Paul Joyce is
cost for bacteria to acquire and maintain resistance to
also funded by the National Science Foundation (NSF DEB-0089756
drugs should be as high as possible, and that models
and NSF DMS-0072198).
can be used to estimate this cost.
We realize that our model simulates the population
dynamics only under the conditions of the evolution
experiment, and that predictions made for time points
Andersson, D. I., and D. Hughes, 1996 Muller's ratchet decreases
far away from our experimental data should be interpre-
fitness of a DNA-based microbe. Proc. Natl. Acad. Sci. USA 93:
ted with caution. However, as stated by Bruce Levin
Andersson, D. I., and B. R. Levin, 1999 The biological cost of
(Lenski 1997, p. 149), "mathematical models with em-
antibiotic resistance. Curr. Opin. Microbiol. 2: 489–493.
pirical estimates of their parameters" are the only way
Austin, D. J., K. G. Kristinsson and R. M. Anderson, 1999 The
"to predict the rate of descent of resistance genes and
relationship between the volume of antimicrobial consumption
in human communities and the frequency of resistance. Proc.
plasmids" and "should be employed to develop antibiot-
Natl. Acad. Sci. USA 96: 1152–1156.
ics use policy" instead of "irresponsible non-quantitative
Bain, L. J., and B. Engelhardt, 1991 Introduction to Probability and
yak-yak." Our modeling approach with empirical esti-
Mathematical Statistics. Duxbury Press, Belmont, CA.
Bergstrom, C. T., M. Lipsitch and B. R. Levin, 2000 Natural selec-
mates of parameters is a step in this direction that is
tion, infectious transfer and the existence conditions for bacterial
unique among the studies that have observed sweeps of
plasmids. Genetics 155: 1505–1519.
antibiotic-sensitive mutants under laboratory conditions
Bi, X., and L. F. Liu, 1996 A replication model for DNA recombina-
tion between direct repeats. J. Mol. Biol. 256: 849–858.
(Godwin and Slater 1979; Modi et al. 1991; Turner
Bickel, P. J., and K. A. Doksum, 1977 Mathematical Statistics: Basic
et al. 1998; Dahlberg and Chao 2003).
Ideas and Selected Topics. Holden-Day, Oakland, CA.
We aim to expand our methodology of modeling
Bjorkman, J., and D. I. Anderson, 2000 The cost of antibiotic resis-
tance from a bacterial perspective. Drug Resist. Upd. 3: 237–245.
and testing to study other experimental evolutionary
Bonhoeffer, S., M. Lipsitch and B. R. Levin, 1997 Evaluating
systems. To do so we will consider other factors that may
treatment protocols to prevent antibiotic resistance. Proc. Natl.
affect the evolutionary process and are unaccounted for
Acad. Sci. USA 94: 12106–12111.
Bo¨ttger, E. C., B. Springer, M. Pletschette and P. Sander, 1998
in our current modeling, such as compensatory muta-
Fitness of antibiotic-resistant microorganisms and compensatory
tions (Bo¨ttger et al. 1998; Bjorkman and Anderson
mutations. Nat. Med. 4: 1343–1344.
2000; Levin et al. 2000; Normark and Normark 2002),
Bouma, J. E., and R. E. Lenski, 1988 Evolution of a bacteria/plasmid
association. Nature 335: 351–352.
background selection on the host and/or on the plas-
Cohen, M. L., 1992 Epidemiology of drug resistance: implications
mid, plasmid loss, and horizontal plasmid transfer. With
for a post-antimicrobial era. Science 257: 1050–1055.
Dahlberg, C., and L. Chao, 2003 Amelioration of the cost of conju-
our current model these effects would likely add noise
gative plasmid carriage in Escherichia coli K12. Genetics 165: 1641–
across lines in other experiments. A statistical test to
detect this added noise is included in the user manual
Dianov, G. L., A. V. Kuzminov, A. V. Mazin and R. I. Salganik,
1991 Molecular mechanisms of deletion formation in Esche-
for our program.
richia coli plasmids. I. Deletion formation mediated by long direct
In conclusion, we have shown that a tetracycline-sensi-
repeats. Mol. Gen. Genet. 228: 153–159.
tive subpopulation of E. coli K12(pB10) emerged during
Drake, J. W., 1991 A constant rate of spontaneous mutation in DNA-
based microbes. Proc. Natl. Acad. Sci. USA 88: 7160–7164.
evolution in an antibiotic-free medium due to deletion
Drake, J. W., B. Charlesworth, D. Charlesworth and J. F. Crow,
of the tet operon. A mathematical model helped explain
1998 Rates of spontaneous mutation. Genetics 148: 1667–1686.
the population dynamics, estimating a high mutation
Dro¨ge, M., A. Pu¨hler and W. Selbitschka, 2000 Phenotypic and
molecular characterization of conjugative antibiotic resistance
rate and a low but significant selection coefficient, and
plasmids isolated from bacterial communities of activated sludge.
allowed us to make long-term predictions regarding the
Mol. Gen. Genet. 263: 471–482.
elimination of antibiotic resistance in the population.
Efron, B., and R. J. Tibshirani, 1993 An Introduction to the Bootstrap.
Chapman & Hall, New York.
The mathematical modeling and statistical analyses thus
Enderle, P. J., and M. A. Farwell, 1998 Electroporation of freshly
worked in perfect symbiosis with the experimental as-
plated Escherichia coli and Pseudomonas aeruginosa cells. BioTech-
pects of this study. The predictions call into question
niques 25: 954–958.
Fisher, R. A., 1922 On the mathematical foundations of theoretical
the general effectiveness of drug cycling as a strategy
statistics. Philos. Trans. R. Soc. Lond. Ser. A 222: 309–368.
to eradicate resistance. Although we do not want our
Flores, M., P. Mavingui, X. Perret, W. J. Broughton, D. Romero
observations to be interpreted as arguments for the futil-
et al., 2000 Prediction, identification, and artificial selection of
DNA rearrangements in Rhizobium : toward a natural genomic
ity of reduced and more prudent drug use, we caution
design. Proc. Natl. Acad. Sci. USA 97: 9138–9143.
against too much optimism regarding eliminating resis-
Gibbons, A., 1992 Exploring new strategies to fight drug-resistant
tance to antibiotics by drug cycling.
microbes. Science 257: 1036–1038.
Godwin, D., and J. H. Slater, 1979 The influence of the growth
We thank two anonymous reviewers for useful comments and sug-
environment on the stability of a drug resistance plasmid in
gestions. We thank Holger Heuer for the primer design and Mayee
Escherichia coli K12. J. Gen. Microbiol. 111: 201–210.
Wong for help with sequencing. Furthermore, we thank Stacey Poler,
Heinemann, J. A., R. G. Ankenbauer and C. F. Amabile-Cuevas,
Whitney Weibler, Erin Quinn, and Monica Flory for their technical
2000 Do antibiotics maintain antibiotic resistance? Drug Discov.
assistance. This publication was made possible by National Institutes
Today 5: 195–204.
L. De Gelder et al.
Helling, R. B., T. Kinney and J. Adams, 1981 The maintenance
Tn10 tetracycline-resistance operon on the fitness of Escherichia
of plasmid-containing organisms in populations of Escherichia coli.
coli K12. Mol. Biol. Evol. 6: 213–225.
J. Gen. Microbiol. 123: 129–141.
Normark, B. H., and S. Normark, 2002 Evolution and spread of
Johnson, T., and N. H. Barton, 2002 The effect of deleterious
antibiotic resistance. J. Intern. Med. 252: 91–106.
alleles on adaptation in asexual populations. Genetics 162: 395–
OTA, 1995 Impacts of Antibiotic-Resistant Bacteria. Office of Technol-
ogy Assessment, United States Congress, Washington, DC.
Joyce, P., Z. Abdo, J. Ponciano, L. De Gelder, L. J. Forney et al.,
Otto, S. P., and M. C. Whitlock, 1997 The probability of fixation
2005 Modeling the impact of periodic bottlenecks, unidirec-
in populations of changing size. Genetics 146: 723–733.
tional mutation, and observational error in experimental evolu-
Rice, J. A., 1995 Mathematical Statistics and Data Analysis. Duxbury
tion. J. Math. Biol. (in press).
Press, Belmont, CA.
Lee, S. W., and G. Edlin, 1985 Expression of tetracycline resistance
Ross, K. A., 1991 Elementary Analysis: The Theory of Calculus. Springer-
in pBR322 derivatives reduces the reproductive fitness of plasmid-
Verlag, New York.
containing Escherichia coli. Gene 39: 173–180.
Sambrook, J., and D. W. Russel, 2001 Molecular Cloning: A Laboratory
Lenski, R. E., 1997 The cost of antibiotic resistance—from the per-
Manual. Cold Spring Harbor Laboratory Press, Cold Spring Har-
spective of the bacterium, pp. 131–151 in Antibiotic Resistance:
Origins, Evolution, Selection and Spread, edited by S. B. Levy. John
Schlu¨ter, A., H. Heuer, R. Szczepanowski, L. J. Forney, C. M.
Wiley & Sons, Chichester, UK.
Thomas et al., 2003 The 64,508 bp IncP-1B antibiotic multiresis-
Lenski, R. E., M. R. Rose, S. C. Simpson and S. C. Tadler, 1991
tance plasmid pB10 isolated from a wastewater treatment plant
Long-term experimental evolution in Escherichia coli. I. Adaptation
provides evidence for recombination between members of differ-
and divergence during 2,000 generations. Am. Nat. 138: 1315–
ent branches of the IncP-1B group. Microbiology 149: 3139–3153.
Schrag, S. J., V. Perrot and B. R. Levin, 1997 Adaptation to the
Lenski, R. E., S. C. Simpson and T. T. Nguyen, 1994 Genetic analysis
fitness costs of antibiotic resistance in Escherichia coli. Proc. R.
of a plasmid-encoded, host genotype-specific enhancement of
Soc. Lond. Ser. B 264: 1287–1291.
bacterial fitness. J. Bacteriol. 176: 3140–3147.
Self, S. G., and K. Liang, 1987 Asymptotic properties of maximum
Levin, B. R., 2001 Minimizing potential resistance: a population
likelihood estimation and likelihood tests under nonstandard
dynamics view. Clin. Infect. Dis. 33 (Suppl 3): S161–S169.
conditions. J. Am. Stat. Assoc. 398: 605–610.
Levin, B. R., M. Lipsitch, V. Perrot, S. J. Schrag, R. Antia et
Spratt, B. G., 1996 Antibiotic resistance: counting the cost. Curr.
Biol. 6: 1219–1221.
al., 1997 The population genetics of antibiotic resistance. Clin.
Stanier, R. Y., N. J. Palleroni and M. Doudoroff, 1966 The aero-
Infect. Dis. 24 (Suppl 1): S9–S16.
bic pseudomonads: a taxonomic study. J. Gen. Microbiol. 43:
Levin, B. R., V. Perrot and N. Walker, 2000 Compensatory muta-
tions, antibiotic resistance and the population genetics of adap-
Thomas, C. M., 2004 Evolution and population genetics of bacterial
tive evolution in bacteria. Genetics 154: 985–997.
plasmids, pp. 509–528 in Plasmid Biology, edited by B. E. Funnell
Levy, S. B., 1994 Balancing the drug-resistance equation. Trends
and G. J. Phillips. ASM Press, Washington, DC.
Microbiol. 2: 341–342.
Turner, P. E., V. S. Cooper and R. E. Lenski, 1998 Tradeoff between
Lovett, S. T., T. J. Gluckman, P. J. Simon, V. A. Sutera, Jr. and
horizontal and vertical modes of transmission in bacterial plas-
P. T. Drapkin, 1994 Recombination between repeats in Esche-
mids. Evolution 52: 315–329.
richia coli by a recA-independent, proximity-sensitive mechanism.
Wahl, L. M., and P. J. Gerrish, 2001 The probability that beneficial
Mol. Gen. Genet. 245: 294–300.
mutations are lost in populations with periodic bottlenecks. Evo-
Martiel, J. L., 2002 Transposable elements and fitness of bacteria.
lution 55: 2606–2610.
Theor. Popul. Biol. 61: 509–518.
Wahl, L. M., and D. C. Krakauer, 2000 Models of experimental
Mazin, A. V., A. V. Kuzminov, G. L. Dianov and R. I. Salganik,
evolution: the role of genetic chance and selective necessity.
1991 Molecular mechanisms of deletion formation in Esche-
Genetics 156: 1437–1448.
richia coli plasmids. II. Deletions mediated by short direct repeats.
Wahl, L. M., P. J. Gerrish and I. Saika-Voivod, 2002 Evaluating
Mol. Gen. Genet. 228: 209–214.
the impact of population bottlenecks in experimental evolution.
Modi, R. I., and J. Adams, 1991 Coevolution in bacterial-plasmid
Genetics 162: 961–971.
populations. Evolution 45: 656–667.
WHO, 1995 Scientific working group on monitoring and manage-
Modi, R. I., C. M. Wilke, R. F. Rosenzweig and J. Adams, 1991 Plas-
ment of bacterial resistance of antimicrobial agents. WHO/CDS/
mid macro-evolution: selection of deletions during adaptation in
BVI 7, World Health Organization, Geneva.
a nutrient-limited environment. Genetica 84: 195–202.
WHO, 2003 Impact of antimicrobial growth promoter termination
Nelder, J. A., and R. Mead, 1965 A simplex algorithm for function
in Denmark. WHO/CDS/CPE/ZFK/2003.1, World Health Orga-
minimization. Comput. J. 7: 308–313.
nization, Geneva.
Nguyen, T. N. M., Q. G. Phan, L. P. Duong, K. P. Bertrand and
R. E. Lenski, 1989 Effects of carriage and expression of the
Communicating editor: H. Ochman
APPENDIX A: IMPORTANT RECURSION
appendix b relies heavily on the solution of the following recursion in deriving the models used in this article:
zt " azt$1 ) cbt$1 ) d.
It follows by induction that
z1 " az0 ) c ) dz2 " az1 ) cb ) d " a2z0 ) (a ) b)c ) (a ) 1)dz3 " az2 ) cb2 ) d " a3z0 ) (a2 ) ab ) b2)c ) (a2 ) a ) 1)dz4 " az3 ) cb3 ) d " a4z0 ) (a3 ) a2b ) ab2 ) b3)c ) (a3 ) a2 ) a ) 1)d,
Modeling Antibiotic Resistance Loss
zt " atz0 ) cat$1 & #b$i ) d&ai.
Using a geometric series identity (Ross 1991, p. 69, Equation 1) results in
at $ bt
zt " atz0 ) c
a $ b
APPENDIX B: DERIVATION OF EQUATIONS 6, 7, AND 8
Let the average number of mutants at a certain time t be mt and the average of the remaining nonmutant
individuals in period t be nt. Therefore, the total population at time t is the sum of mt and nt. We relist Equations
1, 2, and 3 for convenience:
mt " 21)% mt$1 ) 2!nt$1
nt " 2(1 $ !)nt$1
t(*0 , %, !) "
mt ) nt
To solve Equation 3, which represents the proportion of mutants at time t, we need to solve the recursions inEquations 1 and 2. The solution of Equation 2 is obvious:
nt " (2(1 $ !))t n0.
To solve Equation 1 we start by substituting Equation B1 in it to get
mt " 21)%mt$1 ) 2!(2(1 $ !))t$1 n0.
Note that this is the same format as that of Equation A1 with a " 21)%, b " 2(1 $ !), and c " 2!n0. Therefore, we
use Equation A2 to solve Equation B2:
2t(1)%) $ (2(1 $ !))t
2t% $ (1 $ !)t
t " 2t (1)%)m0 ) 2!n0
" 2t %2t%m
Using Equations B1 and B3 we can now solve Equation 3. We first note that
t(*0 , %, !) "
mt ) nt
mt ) nt
2t(1 $ !)t n
t(*0 , %, !) " 1 $
2t[2t%m0 ) !n0((2t% $ (1 $ !)t)/(2% $ (1 $ !))) ) n0(1 $ !)t]
(1 $ !)t n
t(*0 , %, !) " 1 $
2t%m0(2% $ (1 $ !)) ) !n0(2t% $ (1 $ !)t) ) n0(1 $ !)t(2% $ (1 $ !))
Noting that *0 " m0/(m0 ) n0), by definition, results in
(1 $ !)t (1 $ *
t(*0 , %, !) " 1 $
2t%*0(2% $ (1 $ !)) ) !(1 $ *0)(2t% $ (1 $ !)t) ) (1 $ !)t(1 $ *0)(2% $ (1 $ !))
Rearranging again,
0(2% $ (1 $ !)) ) !(1 $ *0 )(2t % $ (1 $ !)t )
t(*0 , %, !) "
2t%*0(2% $ (1 $ !)) ) !(1 $ *0)(2t% $ (1 $ !)t) ) (1 $ !)t(1 $ *0)(2% $ (1 $ !))
which is equivalent to Equation 6 when replacing t with lk.
L. De Gelder et al.
APPENDIX C: DERIVATION OF THE ABSOLUTE GOODNESS-OF-FIT LIKELIHOOD-RATIO TEST STATISTIC
The absolute-best Poisson model fits the data exactly and, hence, exactly predicts the number of mutants in a
sample. Such a model has a parameter for each replicate, i, and at each cycle we sample, k. We call this parameter(ik, corresponding to the expected number of mutants in the current replicate i at the kth cycle. The likelihood-
ratio test statistic . associated with this absolute goodness-of-fit test is
k!K "rki"1(sik )sik e$sik/sik !
C2 holds as the maximum for the numerator is attained at the maximum-likelihood estimate *ˆlk, and the maximum
for the denominator holds when the data are predicted exactly. Some algebraic manipulation results in Equa-tion 10:
. " " "#Dk*ˆlk$sike$Dk*ˆlk)sik .
Source: http://people.ibest.uidaho.edu/~etop/publications/DeGelderGenetics04.pdf
TRAINING ACTIVITIES FOR ACTIVIDADES DE ENTRENAMIENTO DE HABILIDADES VISO-PERCEPTIVAS Visual-Spatial Relationships (Basic Level) Relaciones Viso-espaciales (Nivel Básico) Vidal-López, Joaquín Muiños-Durán, Mónica Codina-Fossas, Marta García-Montero, María Gimeno-Galindo, Patricia TRAINING ACTIVITIES FOR ACTIVIDADES DE ENTRENAMIENTO
DIE ZEHN GEBOTE RATSCHLÄGE FÜR PATIENTEN NACH HERZINFARKT Sie haben vor kurzer Zeit einen Herzinfarkt erlebt und sind nach der Klinik und vielleicht auch nach der Rehabilitation wieder nach Hause und in die gewohnte Umgebung zurückgekehrt. Für die meisten Myokardinfarktpatienten ist nach wenigen Wochen ein nahezu normales Leben, fast wie vor dem Infarkt, möglich. Einige Patienten werden sich aber für die Zukunft Einschränkungen auferlegen müssen, wollen sie sich eine normale Lebenserwartung zurückgewinnen. Dies wird natürlich ihr zukünftiges Leben verändern. Bedenken Sie, dass der frühere amerikanische Präsident Johnson erst nach seinem Herzinfarkt Präsident der Vereinigten Staaten von Amerika geworden ist und viele andere Menschen trotz eines vorangegangenen Infarktes ein für die Gesellschaft und für sie selbst wertvolles und erfülltes Leben gestalten. All diesen Menschen ging es nach dem Herzinfarkt nicht besser, als es Ihnen jetzt ergeht. Man kann und soll also voll Hoffnung sein.